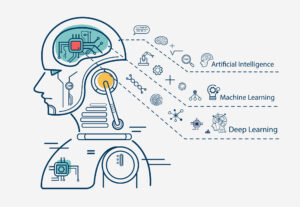
It was in the early 1950s that computational models were first used in the scientific community. As a pioneer in computer games and artificial intelligence, the phrase “Machine Learning” was invented in 1959 by Arthur Samuels, an American pioneer. Thereafter, the idea of Machine Learning began to take shape from a theoretical concept to actual practice.
Always, and for as long as anybody can remember, the ultimate goal of machine learning has always been to allow machines the ability to learn from a variety of data sets without having to be explicitly taught how. Rather than relying on a predetermined code, a machine’s decisions are based on its own experience. Machine learning and Artificial Intelligence are concepts that are designed to manage data recognition, understanding, and analysis.
Throughout history, these conceptions have undergone significant change. Technology has frequently been a stumbling block to further advancement. For a long time, computational infrastructures that could handle the concurrent processing of complicated mathematical tasks through the application of advanced algorithms were required for Machine Learning (and in particular, its subset known as “Deep Learning”).
There were just a few positive outcomes from these efforts. Thus, the so-called “AI Winter” began, in which the industry went through a period of diminished financing and interest in AI. Since its inception in 1984, the phrase has been used to describe a public debate about funding cuts that led to the loss of meaningful research.
In the early 2000s, interest in Machine Learning began to rise, with the development of the Torch software library (2002) and ImageNet (2003)…. (2009). The emergence of a “AI Spring” as the antithesis of a “AI Winter” began in early 2010 AI and Machine Learning have the potential to be a global industry boom, and we are now in the latter stages of that transition in 2018.
Traditionally, these systems have been organized in the following hierarchical fashion: A subset of AI is Machine Learning, which is itself a subset of Deep Learning.
Before vast amounts of digital data and processing power became readily available in the previous decade, classical AI (i.e., rule-based, knowledge-based systems like the Deep Blue chess computer) came to a standstill. Thus, algorithms that require a lot of computer power can now learn from data. This is when ML and DL started to shine and were positioned to revolutionize several industries in terms of how things are done now.
Machine Learning and Deep Learning are currently providing a competitive advantage in the following industries: healthcare, manufacturing, and financial services.
Robotics
When it comes to the supply chain business as a whole, robotics has a huge impact. The usage of Deep Learning applications has already brought automation to a new degree of excellence. In the current manufacturing industry, robots’ abilities to create, manipulate and categorize, sort, and move goods are essential. Drones and autonomous vehicles are employed in the military, public safety, transportation, entertainment, agriculture, and even medical for a variety of purposes. More and more companies are using AI and robotics in their processes.
One of the most important industries relies on AI and robots in space exploration. Although NASA is not a branch of the US military, it is nonetheless a government agency and hence an independent branch. There are numerous technological advancements, including those involving robotics, in use before they become widely known. There is no better example of the convergence of science, technology, industry, and power than space exploration.
Marketing
For the marketing business, Deep Machine Learning is truly reshaping the landscape. Every marketer’s goal is to keep their customers interested, entertained, and informed. The potential applications of deep learning are nearly limitless. As a result, it enables not just better comprehension and target recognition, but also unique and personalized encounters based on behavioral patterns and timely possibilities.
GPU Machine Learning can disrupt businesses and industries by finding potential customers and calculating the best time for a customer to buy. Deep Learning is already a notable example of how technology may help businesses maximize potential while reducing expenses. Predictions and real-time bidding in the ad tech business, for example, utilize Deep Learning to discover and optimize opportunities, as well as to drastically enhance the efficiency of their costs.
Healthcare
As one of the largest fields of knowledge, data, variables, statistics, and unknowns are managed in the health care industry. Instead of just facts, the health and well-being of ourselves and our loved ones are at stake. Because of these high stakes, the medical industry has long been one of the most crucial industries for AI applications to demonstrate and prove their value.
An AI system that is well-trained and adaptable can analyze seemingly insurmountable data sets and countless records to identify trends and tendencies, based on limitless variations of medical records, disease patterns, genetic tendencies, and so on, and this is precisely the benefit of having an AI system. If you want to find remedies for ailments or speed up life-saving discoveries, consider analyzing the DNA of the human body. Medicine will soon be able to provide us with a wide range of real-world applications, such as these.
This field is just beginning to realize the full potential of artificial intelligence (AI), even as many of these applications are being developed and used by clinicians and researchers alike. We are constantly breaking new performance barriers thanks to the tireless work of scientists, developers, and hardware makers. Our human well-being will grow exponentially as a result of GPU manufacturers like NVIDIA and Intel providing the hardware building blocks needed to accomplish better, quicker, and more precise results.
Retail
AI has been a part of the retail business for a long time. Large amounts of computational data are becoming increasingly important in a field that frequently struggles to stay afloat, from maintaining inventory levels to tracking foot activity. Think about the future of retail for a second.
For example, imagine having cameras put in every aisle, with AI image recognition, and scanning capabilities that allow registered consumers to pick up things and exit the store without ever going through a register. A customer’s credit card would be automatically charged for any purchases they made.
For long-term cost savings and unparalleled customer convenience, many retailers are experimenting with this form of AI application. There is also an approach to customer retention, as well as product placement to increase foot traffic.
Autonomous Vehicles
It’s long been known that ride-sharing companies like Uber and Lyft need driverless vehicles in order to extend their platform. In light of recent news about Google’s Waymo initiative and Apple’s (admittedly secret) “Project Titan,” even tech heavyweights like Google and Apple are considering driverless vehicles as a viable option. With Tesla’s “Autopilot” capabilities, big automakers are dipping their toes into the water as well, with automation advancements like these. While achieving complete autonomy in transportation is a tall goal, many people are avidly working toward it.
It’s impossible to deny that we’ll soon be surrounded by self-driving cars for both personal and commercial use, despite the many hurdles this industry faces, from technological impediments to government regulation. A self-driving, automated future will be the norm in the years ahead, despite the fact that we’re not there yet.
Financial Sector
Fraud detection and prevention is one of the banking and payment providers’ most important and public-facing hazards. GPU Deep Learning and Artificial Intelligence platforms help predict economic and corporate growth prospects to make and/or recommend solid investment advice that reduces risk. It is not only necessary but also impossible, to have a system that continuously monitors the whole industry for pattern-building tendencies. With the help of AI and DL, companies have a much better chance of disrupting established industry leaders.
Bit Data
It is becoming increasingly important to make corporate and political decisions based on data mining, statistical analysis, and forecasting, all of which have a wide range of applications. For AI, and its prediction algorithms, having access to historical and real-time data at a rapid rate allows decision-makers in various sectors to make better educated and calculated judgments. (personal human biases aside).
With vast datasets, AI can assist in predicting outcomes and recognizing patterns, but human decision-making is ultimately responsible for all repercussions. These negative outcomes can be mitigated to a greater or lesser extent by the use of an AI system that has been properly trained.
Job Recruitment
HR is a great illustration of how AI and DL may help a sector that is overburdened in some way. For years, companies like LinkedIn and GlassDoor have been using this technology. As a business owner in the current world, sifting through professional profiles to find relevant skills and expertise is a need.
Those days of submitting one’s CV to a panel of peers for approval have long since passed. Based on your social media and online presence as well as your profile’s behavioral patterns, algorithms will now have an even more accurate image of your experience and personality (age, gender, location, etc.). To determine whether or not you’ll be hired, you’ll be compared to other candidates based on your interests, as well as how frequently you change employment.
Automated and algorithmic recruiting has a major benefit over recruiters’ human preferences and biases, even if it initially appears intrusive. DL and AI are going to be the driving forces behind HR in the future.
GPU Machine Learning
Several years ago, a major breakthrough in the field of machine and deep learning technology was made. Created: The GPU Server (Graphics Processing Unit). Deep Learning tasks, which require thousands of CPU cores to do simple computations in parallel, are not suited for CPUs because of their low performance and high cost. For these kinds of tasks, general-purpose CPUs are prohibitively expensive, which is why the graphics processing unit (GPU) was invented.
Because of their design and vast parallel processing capabilities, GPUs were originally designed for image rendering and manipulation. However, developers soon recognized that they could be used for a variety of other activities. These GPUs immediately became the cornerstone for today’s Deep Learning applications because of their enormous number of basic cores.
Hardware makers such as NVIDIA hopped on the bandwagon, with developers and data scientists eager to take advantage. Deep Learning frameworks, apps, and libraries are now supported by an extensive software ecosystem thanks to firms like NVIDIA. Because of GPUs’ many processing cores and ability to handle enormous numbers of simultaneous instructions, graphics processing units (GPUs) shine. Because general-purpose CPUs are so cost-effective, they can be easily distributed as components for Inference and support GPUs in the network and storage sections of computing tasks. Today’s vast and ever-increasing need for infrastructure-parallel processing capabilities is being met in large part by graphics processing units (GPUs).
The need for high-performance GPUs is undeniable, from AI, Machine Learning, Deep Learning, Big Data manipulation, 3D rendering, and even streaming. NVIDIA, a company valued at more than $6.9 billion, has sparked a record-breaking demand for high-performance computing platforms. Deep Learning is estimated to be worth $3.1 billion in 2018 and over $18 billion by 2023, according to market research.
Convolutional Neural networks (CNN) and Recurrent Neural networks (RNN) are two types of deep neural networks that are frequently updated and deployed. As a result, listing them all would be both difficult and risky. However, even while we don’t deal with every network that is constructed, there are some very real use cases that we interact with on a regular basis. Some of the most well-known Deep Learning applications include facial recognition, natural language processing, and voice recognition.
RNNs are better at recognizing patterns in speech and language than CNNs do. Although there are numerous Deep Learning frameworks, some are more suited to certain tasks than others. A sampling of the most common frameworks:
- A well-known deep learning framework, TensorFlow may be used for a variety of natural language processing (NLP) tasks, including speech recognition and text classification. TensorFlow is compatible with both CPUs and GPUs.
- Keras is a Python API-based text generation, categorization, and speech recognition system that works well on both GPUs and CPUs. Tensorflow and Keras are typically deployed together.
- In terms of speech recognition and machine translation, Chainer is the ideal option, as it supports both CNN and DNN networks, as well as NVIDIA CUDA and multi-GPU nodes. Chainer is written in Python.
- It’s no surprise that Pytorch, a popular tool for machine translation, text production, and Natural Language Processing, performs well on GPUs.
- Theano is a Python-based machine learning framework that may be used for text classification, speech recognition, and the creation of new machine learning models.
- As a result of its high performance and speed, CNN relies on Caffe/Caffe2 for image identification. Model Zoo includes a Python interface and a large number of pre-trained models that may be used without creating any code. Caffe/Caffe2 performs well on GPUs and scales effectively as a result of this.
- Text-based data and image/speech recognition are both no problem for CNTK, which is compatible with both CNN and RNN forms of neural networks. It has a Python interface, is multi-node scalable, and performs exceptionally well overall.
- Multiple programming languages and CNN + RNN networks make MXNET an excellent choice for image, audio, and NLP jobs, as well as a highly scalable and GPU-focused solution for these applications.
Conclusions
Unless the robot uprising deters investors for a few years, the field will continue to progress at its current pace if current interests (read: money) are maintained.
Since the human brain is such an incredibly versatile and competent organ, simulating it with software and hardware is a difficult task. Even so, we will continue to improve our technology, develop new Deep Learning approaches, generate more powerful software, and strive toward the ultimate objective of creating a future in which computers can help us advance faster, understand better, and learn quicker.
Is it possible that this will lead to the creation of the singularity? Probably not, but we’ll find out one way or another. Now, we can take advantage of enormous worldwide market opportunities created by AI and Deep Learning, which are geared at making our lives and the world we live in better. Not only are sectors and markets being reshaped, but people’s lives are being transformed as well.
RENT A100 80GB GPU SERVER
GPU server equipped with Intel 24-Core Gold processor is ideal for AI and HPC.