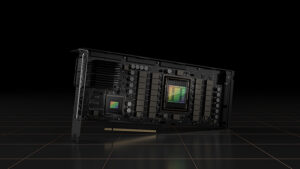
The NVIDIA H100 is based on the Hopper architecture and serves as the “new engine of the world’s artificial intelligence infrastructures.”
AI applications such as speech, conversation, customer service, and recommenders fundamentally reshape data center design.
AI data centers process mountains of continuous data to train and refine AI models. Raw data is ingested, refined, and intelligence is emitted — businesses are manufacturing intelligence and running massive AI factories.
The AI factories operate 24 hours a day and are highly intensive. Minor quality enhancements result in a noticeable increase in customer engagement and company profits.
H100 will assist these factories in operating more efficiently. The “colossal” 80 billion transistor chip is manufactured using TSMC’s 4N process.
Hopper H100 represents the most significant generational leap ever achieved — 9x at-scale training performance over A100 and 30x throughput for large-language model inference.
Hopper is brimming with technological advancements, including a new Transformer Engine that accelerates these networks six times without sacrificing accuracy.
Training for transformer models can be reduced from weeks to days.
NVIDIA has announced that the H100 is in production and available in Q3 2022.
NVIDIA also announced the first discrete data center CPU for high-performance computing, the Grace CPU Superchip.
The Grace CPU Superchip consists of two CPU chips connected via a 900 gigabytes per second NVLink chip-to-chip interconnect to form a 144-core CPU with 1 terabyte per second memory bandwidth.
Grace is the optimal CPU for the world’s artificial intelligence infrastructures.
DGX H100, H100 DGX POD, and DGX SuperPOD will be Hopper GPU-based AI supercomputers.
To connect everything, NVIDIA’s new NVLink high-speed interconnect technology will be integrated into all future NVIDIA chips — CPUs, GPUs, DPUs, and SOCs.
Additionally, NVIDIA will make NVLink available to customers and partners to develop companion chips.
NVLink enables customers to create semi-custom chips and systems that leverage NVIDIA’s platforms and ecosystems.
NVIDIA H100 Specifications
Form Factor | H100 SXM | H100 PCIe |
---|---|---|
FP64 | 30 teraFLOPS | 24 teraFLOPS |
FP64 Tensor Core | 60 teraFLOPS | 48 teraFLOPS |
FP32 | 60 teraFLOPS | 48 teraFLOPS |
TF32 Tensor Core | 1,000 teraFLOPS* | 500 teraFLOPS | 800 teraFLOPS* | 400 teraFLOPS |
BFLOAT16 Tensor Core | 2,000 teraFLOPS* | 1,000 teraFLOPS | 1,600 teraFLOPS* | 800 teraFLOPS |
FP16 Tensor Core | 2,000 teraFLOPS* | 1,000 teraFLOPS | 1,600 teraFLOPS* | 800 teraFLOPS |
FP8 Tensor Core | 4,000 teraFLOPS* | 2,000 teraFLOPS | 3,200 teraFLOPS* | 1,600 teraFLOPS |
INT8 Tensor Core | 4,000 TOPS* | 2,000 TOPS | 3,200 TOPS* | 1,600 TOPS |
GPU memory | 80GB | 80GB |
GPU memory bandwidth | 3TB/s | 2TB/s |
Decoders | 7 NVDEC 7 JPEG | 7 NVDEC 7 JPEG |
Multi-Instance GPUs | Up to 7 MIGS @ 10GB each | Up to 7 MIGS @ 10GB each |
Interconnect | NVLink: 900GB/s PCIe Gen5: 128GB/s | NVLINK: 600GB/s PCIe Gen5: 128GB/s |
Server options | NVIDIA HGX H100 Partner and NVIDIA-Certified Systems with 4 or 8 GPUs NVIDIA DGX H100 with 8 GPUs | NVIDIA-Certified Systems with 1–8 GPUs |
H100 Accelerated Computing
With the NVIDIA H100 Tensor Core GPU, you can get unprecedented performance, scalability, and security for any workload. Up to 256 H100s can be connected to the NVIDIA NVLink Switch System to accelerate exascale workloads and a dedicated Transformer Engine to solve trillion-parameter language models.
To deliver industry-leading conversational AI, H100’s combined technology innovations can speed up large language models by 30X over the previous generation.
Transformational AI Training
NVIDIA H100 GPUs have fourth-generation Tensor Cores and the Transformer Engine with FP8 precision, which provides up to 9X faster training for mixture-of-experts (MoE) models than the previous generation.
The combination of fourth-generation NVlink, which provides 900 GB/s of GPU-to-GPU interconnect; NVLINK Switch System, which accelerates communication by every GPU across nodes; PCIe Gen5; and NVIDIA Magnum IOTM software provides efficient scalability from small businesses to massive, unified GPU clusters.
Exascale high-performance computing (HPC) and trillion-parameter AI are now within reach of all researchers thanks to deploying H100 GPUs at the data center scale, which delivers outstanding performance.
Real-Time Deep Learning Inference
AI uses a diverse set of neural networks to solve various business problems. An excellent AI inference accelerator must be able to accelerate these networks at high speeds and in a variety of ways.
With several advancements accelerating inference by up to 30X and delivering the lowest latency, NVIDIA’s market-leading inference leadership is extended even further. All precisions, including FP64, TF32, FP32, FP16, and INT8, are faster with fourth-generation Tensor Cores. The Transformer Engine uses FP8 and FP16 together to reduce memory usage and increase memory usage performance while maintaining accuracy for large language models.
Data Analytics
In most AI application development projects, data analytics takes up most of the time. Scale-out solutions using commodity CPU-only servers suffer from a lack of scalable computing performance because large datasets are spread across multiple servers.
H100 accelerated servers provide the computing power and 3 terabytes per second (TB/s) of memory bandwidth per GPU and scalability via NVLink and NVSwitch to tackle data analytics with high performance and scale to support massive datasets.
When combined with NVIDIA Quantum-2 Infiniband, Magnum IO software, GPU-accelerated Spark 3.0, and NVIDIA RAPIDSTM, the NVIDIA data center platform is uniquely able to accelerate these massive workloads with unparalleled levels of performance and efficiency.
NVIDIA Confidential Computing
Today’s secure computing solutions are CPU-based, insufficient for compute-intensive workloads such as AI and HPC. The NVIDIA H100 is the world’s first accelerator with confidential computing capabilities thanks to NVIDIA Confidential Computing, a built-in security feature of the NVIDIA HopperTM architecture.
Users can maintain the confidentiality and integrity of their data and applications while taking advantage of the H100 GPUs’ unrivaled acceleration. It establishes a hardware-based trusted execution environment (TEE) that secures and isolates all workloads running on a single H100 GPU, multiple H100 GPUs within a node, or individual MIG instances.
GPU-accelerated applications do not need to be partitioned and can run unchanged within the TEE. Users can combine the power of NVIDIA software for AI and HPC with the security of NVIDIA Confidential Computing’s hardware root of trust.
NVIDIA Grace Hopper
The NVIDIA Grace Hopper CPU+GPU architecture, purpose-built for terabyte-scale accelerated computing and providing 10X higher performance on large-model AI and HPC, will be powered by the Hopper Tensor Core GPU. The NVIDIA Grace CPU takes advantage of the Arm architecture’s flexibility to create a CPU and server architecture explicitly built for accelerated computing.
NVIDIA’s ultra-fast chip-to-chip interconnects the Hopper GPU and the Grace CPU, delivering 900GB/s of bandwidth, 7X faster than PCIe Gen5. Compared to today’s fastest servers, this innovative design will provide up to 30X higher aggregate system memory bandwidth to the GPU and up to 10X higher performance for applications that handle terabytes of data.
Enterprise-Ready Utilization
IT managers strive to maximize compute resource utilization (peak and average) in the data center. They frequently use dynamic compute reconfiguration to appropriately size resources for the workloads.
The H100’s second-generation Multi-Instance GPU (MIG) maximizes GPU utilization by securely partitioning each GPU into seven separate instances. H100’s support for confidential computing enables secure end-to-end multi-tenant usage, making it ideal for cloud service provider (CSP) environments.
Infrastructure managers can use H100 with MIG to standardize their GPU-accelerated infrastructure while also having the flexibility to provide GPU resources with greater granularity, ensuring that developers get the right amount of accelerated compute and get the most out of all of their GPU resources.