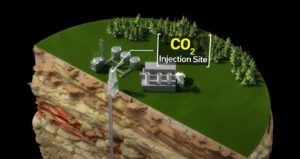
Novel neural operators speed up carbon capture and storage simulations, paving the way for climate change mitigation.
A group of scientists has developed a new artificial intelligence-based tool that can help lock up greenhouse gases like CO2 in porous rock formations faster and more precisely than ever before.
Carbon capture technology, also known as carbon sequestration, is a climate change mitigation technique that involves rerouting CO2 emissions from power plants underground. While doing so, scientists must avoid creating too much pressure in the rock by injecting CO2, which can fracture geological formations and leak carbon into aquifers above the site or even into the atmosphere.
U-FNO, a new neural operator architecture, simulates pressure levels during carbon storage in a fraction of a second while doubling accuracy on certain tasks, assisting scientists in determining the best injection rates and locations. It was revealed this week in a study published in Advances in Water Resources by Stanford University, California Institute of Technology, Purdue University, and NVIDIA, with co-authors from Stanford University, California Institute of Technology, Purdue University, and NVIDIA.
Carbon capture and storage is one of the few options for decarbonizing and reducing emissions in industries like refining, cement, and steel. More than a hundred carbon capture and storage facilities are being built around the world.
ExxonMobil, which funded the study, will use U-FNO to speed up carbon storage predictions.
“Reservoir simulators are intensive computer models used by engineers and scientists to study multiphase flows and other complex physical phenomena in the earth’s subsurface geology,” explained James V. White, ExxonMobil’s subsurface carbon storage manager. “Machine learning techniques like the ones used in this study provide a reliable pathway for quantifying uncertainties in large-scale subsurface flow models like carbon capture and sequestration, allowing for better decision-making.”
How Scientists in the Field of Carbon Storage Make Use of Machine Learning
Carbon storage simulations are used by scientists to determine the best injection sites and rates, control pressure buildup, maximize storage efficiency, and ensure that the injection activity does not fracture the rock formation. Understanding the carbon dioxide plume — the spread of CO2 through the ground — is also critical for a successful storage project.
Traditional carbon sequestration simulators are time-consuming and computationally costly. Machine learning models achieve similar levels of accuracy while requiring significantly less time and money.
U-FNO is a gas saturation and pressure buildup prediction system based on the U-Net neural network and the Fourier neural operator architecture, also known as FNO. U-FNO is twice as accurate as a state-of-the-art convolutional neural network for the task while requiring only a third of the training data.
Anima Anandkumar, director of machine learning research at NVIDIA and Bren professor in the Computing + Mathematical Sciences Department at Caltech, said, “Our machine learning method for scientific modeling is fundamentally different from standard neural networks, where we typically work with images of a fixed resolution.” “We have different resolutions in scientific modeling depending on how and where we sample.” Our model generalizes well across resolutions without requiring retraining, resulting in massive speedups.”
A web application with trained U-FNO models is available to provide real-time predictions for carbon storage projects.
“Recent advances in AI, such as FNOs, can speed up computations by orders of magnitude, paving the way for the scale-up of carbon capture and storage technologies,” said Ranveer Chandra, Microsoft’s managing director of research for industry and a collaborator on the Northern Lights initiative, a full-scale carbon capture, and storage project in Norway. “Using the distributed memory of many NVIDIA Tensor Core GPUs, our model-parallel FNO can scale to realistic 3D problem sizes.”
Novel Neural Operators Accelerate Predictions of CO2 Storage
Scientists can use U-FNO to simulate how pressure levels will rise and where CO2 will spread over the course of 30 years of injection. In comparison to traditional methods, GPU acceleration with U-FNO allows these 30-year simulations to be completed in a hundredth of a second on a single NVIDIA A100 Tensor Core GPU, instead of ten minutes.
Researchers can now simulate a large number of injection sites quickly using GPU-accelerated machine learning. Choosing a site without this tool is like taking a chance.
The U-FNO model is designed to simulate plume migration and pressure during the injection process when there is the greatest risk of exceeding the CO2 injection volume. The Sherlock computing cluster at Stanford used NVIDIA A100 GPUs to develop it.
“To achieve net-zero, we’ll need low-emission energy sources as well as negative-emission technologies like carbon capture and storage,” said Farah Hariri, a collaborator on U-FNO and the technical lead on climate change mitigation projects for NVIDIA’s Earth-2, the world’s first AI digital twin supercomputer. “By applying Fourier neural operators to carbon storage, we demonstrated how AI can aid in the mitigation of climate change.” Earth-2 will make use of those methods.” Earth-2 will tackle climate science challenges and contribute to global climate change mitigation efforts by using FNO-like models.